快速入门#
欢迎使用 Flax!
Flax 是一个基于 JAX 构建的开源 Python 神经网络库。 本教程演示了如何使用 Flax Linen API 构建简单的卷积神经网络 (CNN),并在 MNIST 数据集上训练网络以进行图像分类。
1. 安装 Flax#
!pip install -q flax>=0.7.5
2. 加载数据#
Flax 可以使用任何数据加载管道,此示例演示如何使用 TFDS。 定义一个加载和准备 MNIST 数据集并将样本转换为浮点数的函数。
import tensorflow_datasets as tfds # TFDS for MNIST
import tensorflow as tf # TensorFlow operations
def get_datasets(num_epochs, batch_size):
"""Load MNIST train and test datasets into memory."""
train_ds = tfds.load('mnist', split='train')
test_ds = tfds.load('mnist', split='test')
train_ds = train_ds.map(lambda sample: {'image': tf.cast(sample['image'],
tf.float32) / 255.,
'label': sample['label']}) # normalize train set
test_ds = test_ds.map(lambda sample: {'image': tf.cast(sample['image'],
tf.float32) / 255.,
'label': sample['label']}) # normalize test set
train_ds = train_ds.repeat(num_epochs).shuffle(1024) # create shuffled dataset by allocating a buffer size of 1024 to randomly draw elements from
train_ds = train_ds.batch(batch_size, drop_remainder=True).prefetch(1) # group into batches of batch_size and skip incomplete batch, prefetch the next sample to improve latency
test_ds = test_ds.shuffle(1024) # create shuffled dataset by allocating a buffer size of 1024 to randomly draw elements from
test_ds = test_ds.batch(batch_size, drop_remainder=True).prefetch(1) # group into batches of batch_size and skip incomplete batch, prefetch the next sample to improve latency
return train_ds, test_ds
3. 定义网络#
通过子类化 Flax 模块 使用 Linen API 创建卷积神经网络。 因为此示例中的架构相对简单(你只是堆叠层),你可以直接在 __call__
方法中定义内联子模块,并使用 @compact
装饰器包装它。 要了解有关 Flax Linen @compact
装饰器的更多信息,请参阅 setup
与 compact
指南。
from flax import linen as nn # Linen API
class CNN(nn.Module):
"""A simple CNN model."""
@nn.compact
def __call__(self, x):
x = nn.Conv(features=32, kernel_size=(3, 3))(x)
x = nn.relu(x)
x = nn.avg_pool(x, window_shape=(2, 2), strides=(2, 2))
x = nn.Conv(features=64, kernel_size=(3, 3))(x)
x = nn.relu(x)
x = nn.avg_pool(x, window_shape=(2, 2), strides=(2, 2))
x = x.reshape((x.shape[0], -1)) # flatten
x = nn.Dense(features=256)(x)
x = nn.relu(x)
x = nn.Dense(features=10)(x)
return x
查看模型层#
创建 Flax 模块的实例,并使用 Module.tabulate
方法,通过传递 RNG 密钥和模板图像输入来可视化模型层的表格。
import jax
import jax.numpy as jnp # JAX NumPy
cnn = CNN()
print(cnn.tabulate(jax.random.key(0), jnp.ones((1, 28, 28, 1)),
compute_flops=True, compute_vjp_flops=True))
CNN Summary
┏━━━━━━━━━┳━━━━━━━━┳━━━━━━━━━━━━┳━━━━━━━━━━━┳━━━━━━━━━┳━━━━━━━━━━━┳━━━━━━━━━━━━┓
┃ path ┃ module ┃ inputs ┃ outputs ┃ flops ┃ vjp_flops ┃ params ┃
┡━━━━━━━━━╇━━━━━━━━╇━━━━━━━━━━━━╇━━━━━━━━━━━╇━━━━━━━━━╇━━━━━━━━━━━╇━━━━━━━━━━━━┩
│ │ CNN │ float32[1… │ float32[… │ 8708106 │ 26957556 │ │
├─────────┼────────┼────────────┼───────────┼─────────┼───────────┼────────────┤
│ Conv_0 │ Conv │ float32[1… │ float32[… │ 455424 │ 1341472 │ bias: │
│ │ │ │ │ │ │ float32[3… │
│ │ │ │ │ │ │ kernel: │
│ │ │ │ │ │ │ float32[3… │
│ │ │ │ │ │ │ │
│ │ │ │ │ │ │ 320 (1.3 │
│ │ │ │ │ │ │ KB) │
├─────────┼────────┼────────────┼───────────┼─────────┼───────────┼────────────┤
│ Conv_1 │ Conv │ float32[1… │ float32[… │ 6566144 │ 19704320 │ bias: │
│ │ │ │ │ │ │ float32[6… │
│ │ │ │ │ │ │ kernel: │
│ │ │ │ │ │ │ float32[3… │
│ │ │ │ │ │ │ │
│ │ │ │ │ │ │ 18,496 │
│ │ │ │ │ │ │ (74.0 KB) │
├─────────┼────────┼────────────┼───────────┼─────────┼───────────┼────────────┤
│ Dense_0 │ Dense │ float32[1… │ float32[… │ 1605888 │ 5620224 │ bias: │
│ │ │ │ │ │ │ float32[2… │
│ │ │ │ │ │ │ kernel: │
│ │ │ │ │ │ │ float32[3… │
│ │ │ │ │ │ │ │
│ │ │ │ │ │ │ 803,072 │
│ │ │ │ │ │ │ (3.2 MB) │
├─────────┼────────┼────────────┼───────────┼─────────┼───────────┼────────────┤
│ Dense_1 │ Dense │ float32[1… │ float32[… │ 5130 │ 17940 │ bias: │
│ │ │ │ │ │ │ float32[1… │
│ │ │ │ │ │ │ kernel: │
│ │ │ │ │ │ │ float32[2… │
│ │ │ │ │ │ │ │
│ │ │ │ │ │ │ 2,570 │
│ │ │ │ │ │ │ (10.3 KB) │
├─────────┼────────┼────────────┼───────────┼─────────┼───────────┼────────────┤
│ │ │ │ │ │ Total │ 824,458 │
│ │ │ │ │ │ │ (3.3 MB) │
└─────────┴────────┴────────────┴───────────┴─────────┴───────────┴────────────┘
Total Parameters: 824,458 (3.3 MB)
4. 创建 TrainState
#
Flax 中的一个常见模式是创建一个表示整个训练状态(包括步数、参数和优化器状态)的单个数据类。
由于这是一个常见的模式,Flax 提供了 flax.training.train_state.TrainState
类,该类可以满足大多数基本用例。
!pip install -q clu
from clu import metrics
from flax.training import train_state # Useful dataclass to keep train state
from flax import struct # Flax dataclasses
import optax # Common loss functions and optimizers
我们将使用 clu
库来计算指标。有关 clu
的更多信息,请参阅 repo 和 notebook。
@struct.dataclass
class Metrics(metrics.Collection):
accuracy: metrics.Accuracy
loss: metrics.Average.from_output('loss')
然后,你可以子类化 train_state.TrainState
,使其也包含指标。 这样做的好处是,我们只需要将单个参数传递给诸如 train_step()
(见下文)之类的函数,即可一次性计算损失、更新参数和计算指标。
class TrainState(train_state.TrainState):
metrics: Metrics
def create_train_state(module, rng, learning_rate, momentum):
"""Creates an initial `TrainState`."""
params = module.init(rng, jnp.ones([1, 28, 28, 1]))['params'] # initialize parameters by passing a template image
tx = optax.sgd(learning_rate, momentum)
return TrainState.create(
apply_fn=module.apply, params=params, tx=tx,
metrics=Metrics.empty())
5. 训练步骤#
一个函数,该函数
使用
TrainState.apply_fn
(其中包含Module.apply
方法(前向传播))根据参数和一批输入图像评估神经网络。使用预定义的
optax.softmax_cross_entropy_with_integer_labels()
计算交叉熵损失。 请注意,此函数需要整数标签,因此无需将标签转换为 one-hot 编码。使用
jax.grad
评估损失函数的梯度。将梯度 pytree 应用于优化器,以更新模型的参数。
使用 JAX 的 @jit 装饰器来跟踪整个 train_step
函数,并使用 XLA 将其即时编译为融合设备操作,这些操作在硬件加速器上运行更快、更高效。
@jax.jit
def train_step(state, batch):
"""Train for a single step."""
def loss_fn(params):
logits = state.apply_fn({'params': params}, batch['image'])
loss = optax.softmax_cross_entropy_with_integer_labels(
logits=logits, labels=batch['label']).mean()
return loss
grad_fn = jax.grad(loss_fn)
grads = grad_fn(state.params)
state = state.apply_gradients(grads=grads)
return state
6. 指标计算#
为损失和准确性指标创建单独的函数。 损失使用 optax.softmax_cross_entropy_with_integer_labels
函数计算,而准确性使用 clu.metrics
计算。
@jax.jit
def compute_metrics(*, state, batch):
logits = state.apply_fn({'params': state.params}, batch['image'])
loss = optax.softmax_cross_entropy_with_integer_labels(
logits=logits, labels=batch['label']).mean()
metric_updates = state.metrics.single_from_model_output(
logits=logits, labels=batch['label'], loss=loss)
metrics = state.metrics.merge(metric_updates)
state = state.replace(metrics=metrics)
return state
7. 下载数据#
num_epochs = 10
batch_size = 32
train_ds, test_ds = get_datasets(num_epochs, batch_size)
8. 设置随机种子#
设置 TF 随机种子以确保数据集随机化(使用
tf.data.Dataset.shuffle
)是可重现的。获取一个 PRNGKey 并将其用于参数初始化。(了解有关 JAX PRNG 设计 和 PRNG 链的更多信息。)
tf.random.set_seed(0)
init_rng = jax.random.key(0)
9. 初始化 TrainState
#
请记住,函数 create_train_state
初始化模型参数、优化器和指标,并将它们放入返回的训练状态数据类中。
learning_rate = 0.01
momentum = 0.9
state = create_train_state(cnn, init_rng, learning_rate, momentum)
del init_rng # Must not be used anymore.
10. 训练和评估#
通过以下方式创建“随机”数据集
重复数据集,使其等于训练 epoch 的数量
分配一个大小为 1024 的缓冲区(包含数据集中的前 1024 个样本),从中随机抽取批次
每次从缓冲区中随机抽取一个样本时,都会将数据集中的下一个样本加载到缓冲区中
定义一个训练循环,该循环
从数据集中随机抽取批次。
对每个训练批次运行优化步骤。
计算一个 epoch 中每个批次的平均训练指标。
使用更新后的参数计算测试集的指标。
记录训练和测试指标以进行可视化。
在 10 个 epoch 后完成训练和测试后,输出应显示你的模型能够达到大约 99% 的准确率。
# since train_ds is replicated num_epochs times in get_datasets(), we divide by num_epochs
num_steps_per_epoch = train_ds.cardinality().numpy() // num_epochs
metrics_history = {'train_loss': [],
'train_accuracy': [],
'test_loss': [],
'test_accuracy': []}
for step,batch in enumerate(train_ds.as_numpy_iterator()):
# Run optimization steps over training batches and compute batch metrics
state = train_step(state, batch) # get updated train state (which contains the updated parameters)
state = compute_metrics(state=state, batch=batch) # aggregate batch metrics
if (step+1) % num_steps_per_epoch == 0: # one training epoch has passed
for metric,value in state.metrics.compute().items(): # compute metrics
metrics_history[f'train_{metric}'].append(value) # record metrics
state = state.replace(metrics=state.metrics.empty()) # reset train_metrics for next training epoch
# Compute metrics on the test set after each training epoch
test_state = state
for test_batch in test_ds.as_numpy_iterator():
test_state = compute_metrics(state=test_state, batch=test_batch)
for metric,value in test_state.metrics.compute().items():
metrics_history[f'test_{metric}'].append(value)
print(f"train epoch: {(step+1) // num_steps_per_epoch}, "
f"loss: {metrics_history['train_loss'][-1]}, "
f"accuracy: {metrics_history['train_accuracy'][-1] * 100}")
print(f"test epoch: {(step+1) // num_steps_per_epoch}, "
f"loss: {metrics_history['test_loss'][-1]}, "
f"accuracy: {metrics_history['test_accuracy'][-1] * 100}")
train epoch: 1, loss: 0.20290373265743256, accuracy: 93.87000274658203
test epoch: 1, loss: 0.07591685652732849, accuracy: 97.60617065429688
train epoch: 2, loss: 0.05760224163532257, accuracy: 98.28500366210938
test epoch: 2, loss: 0.050395529717206955, accuracy: 98.3974380493164
train epoch: 3, loss: 0.03897436335682869, accuracy: 98.83000183105469
test epoch: 3, loss: 0.04574578255414963, accuracy: 98.54767608642578
train epoch: 4, loss: 0.028721099719405174, accuracy: 99.15166473388672
test epoch: 4, loss: 0.035722777247428894, accuracy: 98.91827392578125
train epoch: 5, loss: 0.021948494017124176, accuracy: 99.37999725341797
test epoch: 5, loss: 0.035723842680454254, accuracy: 98.87820434570312
train epoch: 6, loss: 0.01705147698521614, accuracy: 99.54833221435547
test epoch: 6, loss: 0.03456473350524902, accuracy: 98.96835327148438
train epoch: 7, loss: 0.014007646590471268, accuracy: 99.6116714477539
test epoch: 7, loss: 0.04089202359318733, accuracy: 98.7880630493164
train epoch: 8, loss: 0.011265480890870094, accuracy: 99.73333740234375
test epoch: 8, loss: 0.03337760642170906, accuracy: 98.93830108642578
train epoch: 9, loss: 0.00918484665453434, accuracy: 99.78334045410156
test epoch: 9, loss: 0.034478139132261276, accuracy: 98.96835327148438
train epoch: 10, loss: 0.007260234095156193, accuracy: 99.84166717529297
test epoch: 10, loss: 0.032822880893945694, accuracy: 99.07852172851562
11. 可视化指标#
import matplotlib.pyplot as plt # Visualization
# Plot loss and accuracy in subplots
fig, (ax1, ax2) = plt.subplots(1, 2, figsize=(15, 5))
ax1.set_title('Loss')
ax2.set_title('Accuracy')
for dataset in ('train','test'):
ax1.plot(metrics_history[f'{dataset}_loss'], label=f'{dataset}_loss')
ax2.plot(metrics_history[f'{dataset}_accuracy'], label=f'{dataset}_accuracy')
ax1.legend()
ax2.legend()
plt.show()
plt.clf()
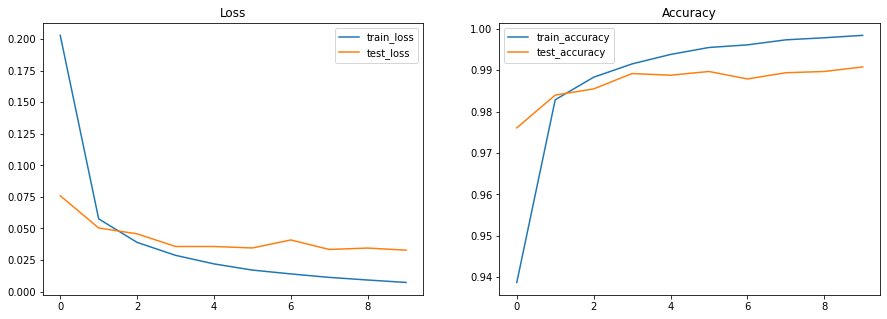
<Figure size 600x400 with 0 Axes>
12. 在测试集上执行推理#
定义一个已 jitted 的推理函数 pred_step
。 使用学习到的参数对测试集进行模型推理,并可视化图像及其对应的预测标签。
@jax.jit
def pred_step(state, batch):
logits = state.apply_fn({'params': state.params}, test_batch['image'])
return logits.argmax(axis=1)
test_batch = test_ds.as_numpy_iterator().next()
pred = pred_step(state, test_batch)
fig, axs = plt.subplots(5, 5, figsize=(12, 12))
for i, ax in enumerate(axs.flatten()):
ax.imshow(test_batch['image'][i, ..., 0], cmap='gray')
ax.set_title(f"label={pred[i]}")
ax.axis('off')
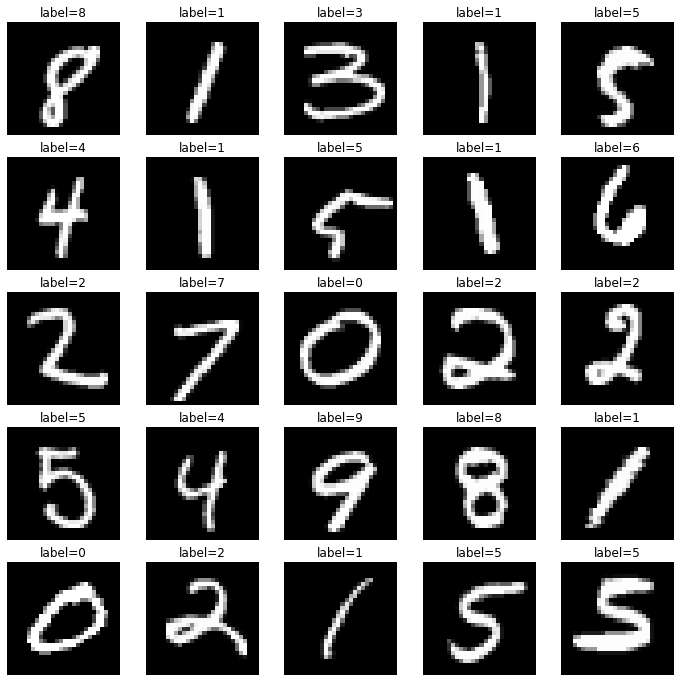
恭喜! 你已到达带注释的 MNIST 示例的结尾。 你可以重新访问同一个示例,但它以不同的方式结构化为几个 Python 模块、测试模块、配置文件、另一个 Colab 以及 Flax Git 仓库中的文档